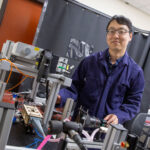
Revolutionizing materials science with use-inspired AI research
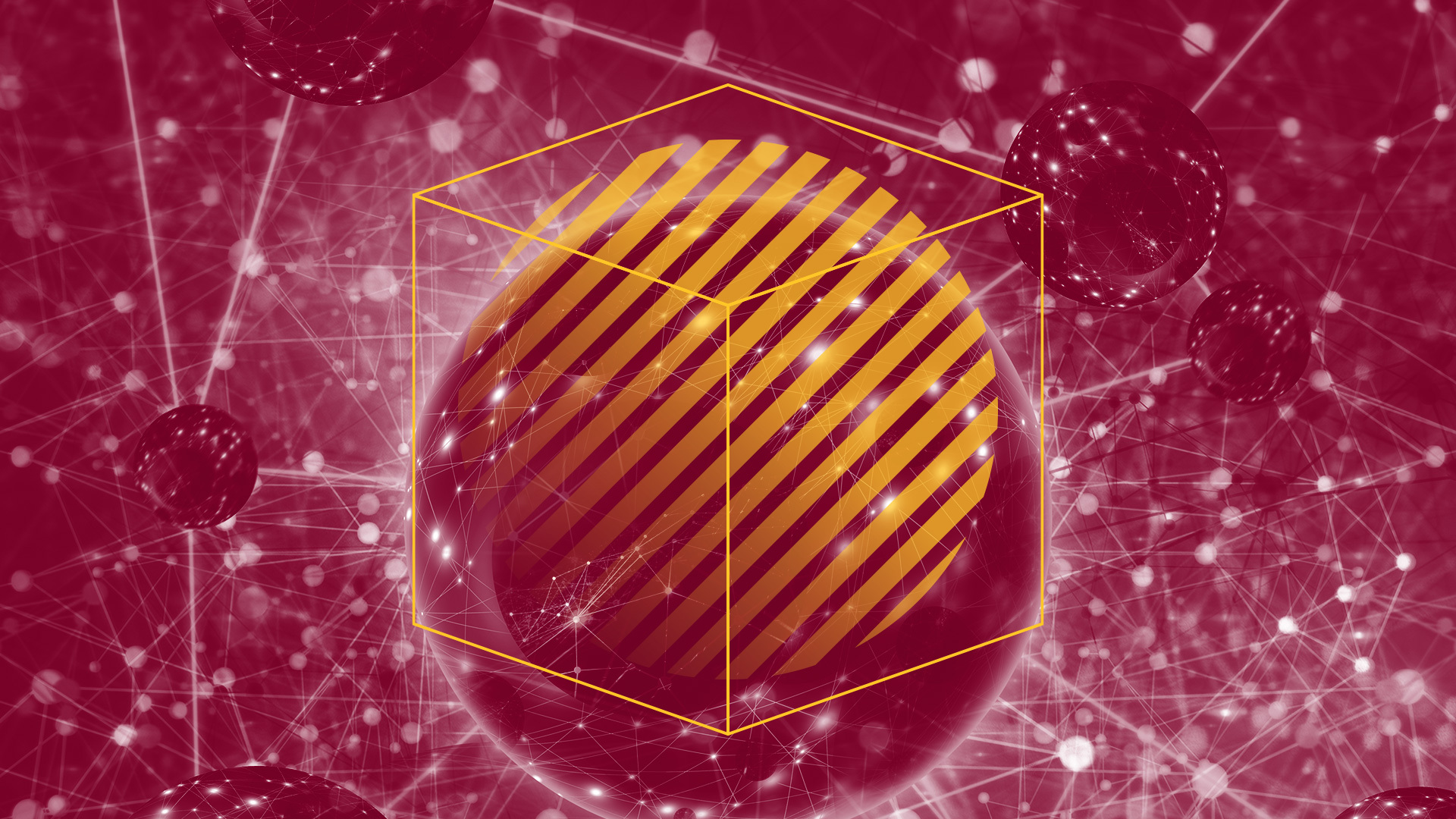
Associate Professor Yang Jiao and Assistant Professor Yi "Max" Ren are working on a two-year project to introduce artificial intelligence to the field of materials science. While traditional materials science involves applying mathematical equations at a limited scope, this new approach will provide a more robust understanding of material behavior. Graphic created by Marc Collins/ASU
As long as materials science has been studied, the field has operated in largely the same way. Researchers form hypotheses and test them using experiments and simulations. From there, they formulate mathematical equations that describe and predict a material’s characteristics and behaviors.
However, with recent innovations and rapid progress in many other aspects of engineering, some materials scientists believe the field is reaching the limits of what can be accomplished using traditional scientific methods.
A multi-institutional team led by Associate Professor Yang Jiao and Assistant Professor Yi “Max” Ren are using machine learning and artificial intelligence to develop a new way to study materials science — a subdiscipline they call artificial intelligence materials science engineering, or AIMSE.
“The overall goal is to revolutionize our way of understanding and discovering materials,” Jiao says.
In a two-year project supported by seed funding from the National Science Foundation, Jiao and Ren plan to establish an AI institute to show how AI can be used to determine properties of material systems from the atomic level to the fourth dimension.
The project will impact materials used in applications ranging from aerospace engineering to soft robotic prosthetics by helping materials scientists and engineers better understand a material’s response to external stimuli.
Jiao and Ren expect the project will develop into a five-year, multi-institutional initiative for AIMSE, for which the Ira A. Fulton Schools of Engineering at Arizona State University will be the lead institution.
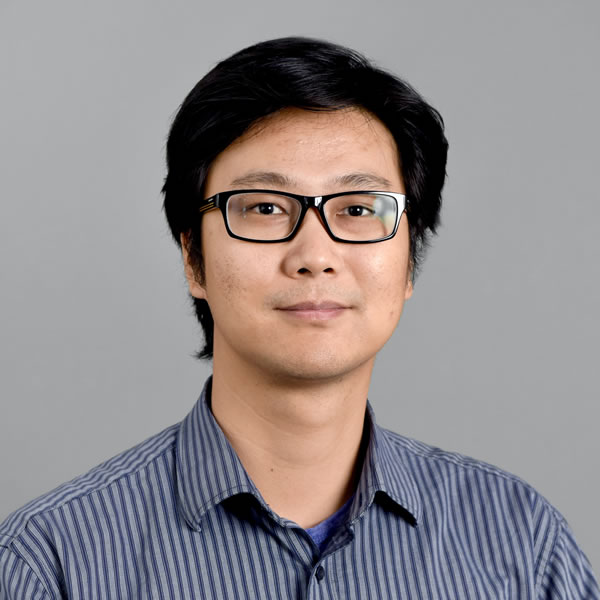
Yang Jiao
Pushing the limits of traditional materials science
Though effective, traditional, equation-based materials science has been found to have limitations that affect the ability of materials scientists and engineers to accurately predict material behavior, especially in extreme conditions.
“The difficulty is that whenever you push the system to the limits, most of the theories that were previously developed may no longer hold true,” Jiao says. “These material theories may only focus on a single phenomenon or a single length scale. They focus on one particular mechanism.”
For example, space shuttles need to be resistant to heat in order to withstand extreme conditions when exiting or re-entering the Earth’s atmosphere. Traditional materials science would suggest that ceramic materials could be aptly used in the space shuttle design.
But while ceramic materials are very heat resistant, they are also very brittle. If there is even a small crack in the ceramic, the entire part will fail, putting the shuttle at risk of catastrophic failure.
“If we want to really understand material behavior in extreme conditions, such as when we need a really strong material in high temperatures, the current method of looking at each property separately is not sufficient,” says Jiao. “We need to consider the whole material system as integrated and consider the interactions and mechanisms coupled with one another starting all the way from the atomic level.”
Material behavior all the way down to the atom
Jiao and Ren are particularly interested in applications of materials in extreme conditions, such as materials for spacecrafts or airplanes, smart materials for soft robotics applications, and materials for nuclear and petroleum engineering.
To design materials that operate in such conditions, Jiao says they have to look at the microstructure of the material that determines its behavior.
For example, he says, placing a material under an optical microscope will allow you to study its finer textures. But if you use an electron microscope, which has much higher magnification capabilities, you can see its individual atoms.
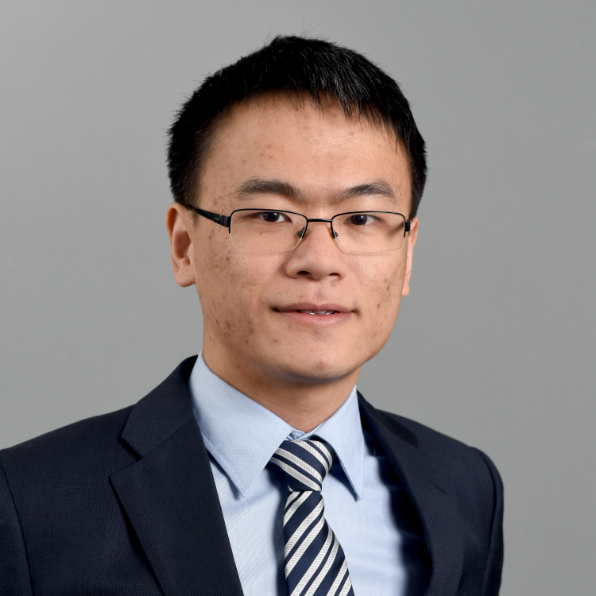
Yi “Max” Ren
These microstructures are characterized by different length scales, which range from the atomic level to the visible structural level. All of these microstructures play an important role in determining the material’s properties, but they cannot all be evaluated at once.
“Currently, when people develop methods and theories, they focus on only one length scale,” Jiao says. “For example, on the atomic length scale, you have the density functional theory. If you look at another length scale there are finite element methods, but these are all separate, individual theories. The challenge now is if you want to correctly predict material behavior in extreme conditions, you have to consider coupling between all these different length scales.”
Jiao explains how applying a force to a material may first affect it visibly on the structural level. However, that force will propagate down all the way to the atomic scale as well.
And if the force is large enough, it can even shear the bonds between atoms and that tear will propagate up to the macroscopic scale eventually, creating a small crack in the structure.
All of these mechanisms are coupled across all length scales, and most current theories do not explicitly consider how changes in one length scale affect changes in another.
“With artificial intelligence, we are not looking at the mathematics at each individual length scale,” Jiao says. “We are looking for an implicit representation of the entire material system in terms of an artificial neural network with a physics-inspired architecture that naturally links all length scales.”
Because the behavior of the material is determined by all of its different microstructures, Jiao and Ren are seeking to represent the key microstructure features similar to the relationships between nodes in a neural network.
This can capture the physical interactions between different atoms, dislocations and other microstructure features in the entire material system.
“Now, if we are to use the well-developed neural network model, we could find new mathematical relationships based on the interactions between these nodes and discover emerging physics and new mechanisms across different length scales,” Jiao says. “From there, we can determine the behavior of the entire material structure while taking all microstructures into account.”
Adding a fourth dimension to materials science
In addition to examining material behavior at different length scales, Jiao and Ren are looking to assess the behavior of materials in extreme environments over time.
“Atoms are vibrating, moving and interacting with one another very quickly,” Jiao says. “But if you look at the actual material as a whole, you do not see these motions. You will see some slow motions, such as a growing crack.”
The different speeds at which microstructures move are referred to as time scales.
“Usually, if you go to smaller length scales, you increase the time scale, because microstructures will move very fast,” Jiao says. “But if you go to the larger length scales, the time scale is decreased as structures move more slowly.”
In 3D materials science, materials are analyzed as if they are static and the mathematical relationships to describe microstructure behavior assume that they do not evolve over time.
The consideration of time is part of what makes this project pioneering in the field of 4D materials science.
“We are interested in the evolution of microstructures that can be part of the key process in determining their properties,” Jiao says. “For example, if you think about a material with a crack, we are interested in seeing how the crack evolves over time under different conditions. This process occurs in a three-dimensional material, but it is a four-dimensional process.”
Jiao and Ren are collaborating with Assistant Professor Kumar Ankit and Assistant Professor Houlong Zhuang at ASU. They’ll also work with Nikhilesh Chawla, the Ransburg Professor of mechanical engineering at Purdue, Wanliang Shan, assistant professor at Syracuse University; Hailong Chen, assistant professor of mechanical engineering at the University of Kentucky; and Pejman Tahmasebi, assistant professor of petroleum engineering at the University of Wyoming.