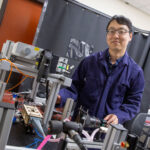
Computing scenarios for defusing polarizing politics
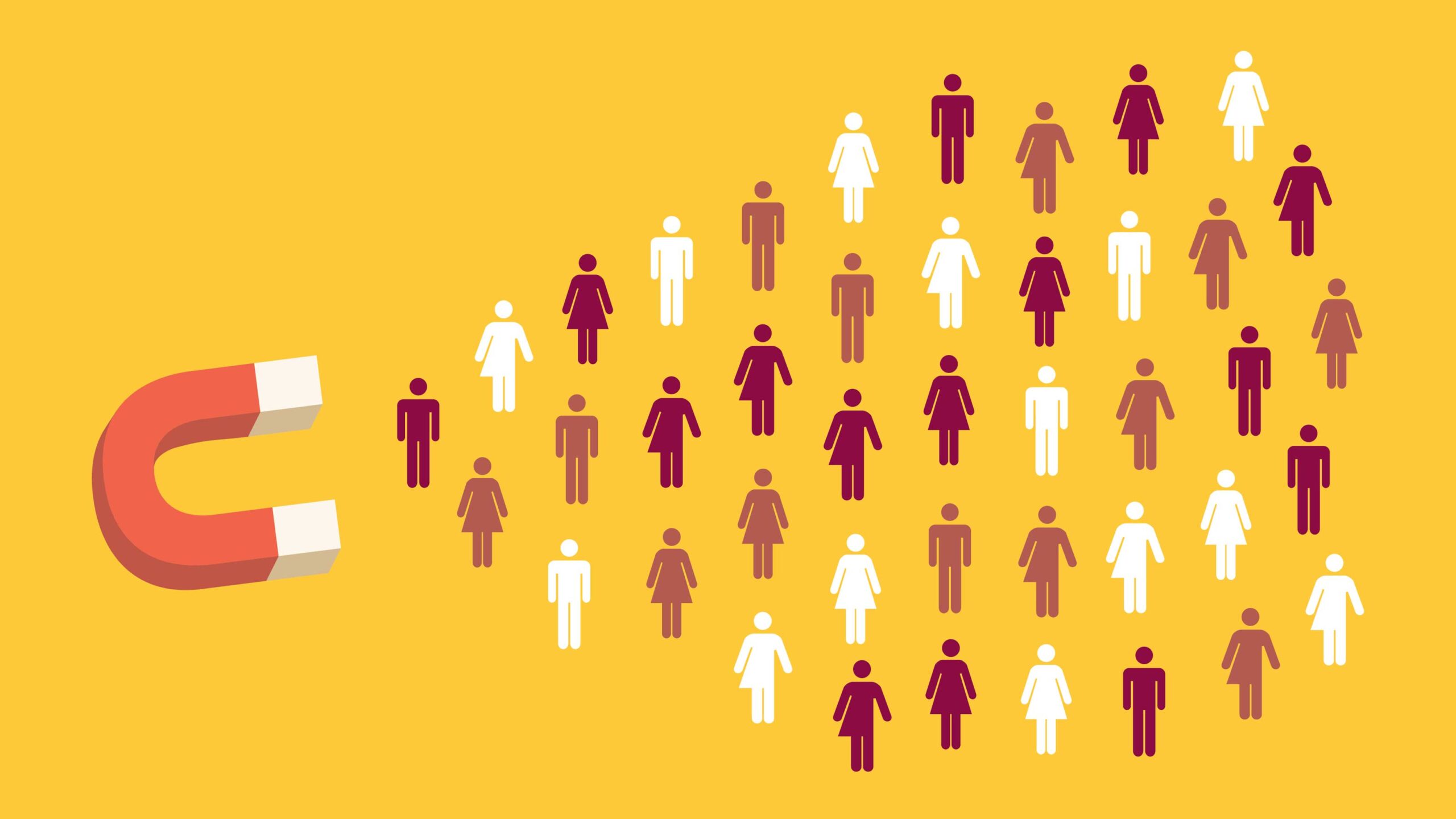
Above: Two computer scientists in the Ira A. Fulton Schools of Engineering and a University of Michigan political scientist teamed up on research exploring ways people at varying points on the political spectrum could be drawn back to unifying societal values and away from deep ideological divides that can erode democracy. Illustration by Rhonda Hitchcock-Mast/ASU and Shutterstock
Opposites may attract when it comes to personal relationships. In political affairs today, however, that claim is becoming more difficult to assert.
New research shows that common ground is shrinking in politics and people on opposite ends of the ideological spectrum are more entrenched in their divergent positions than at any time in recent history.
Those conclusions are not derived only from results of traditional opinion polls. In this era of big data and cutting-edge computational techniques, scientists are modeling patterns of various human interactions to examine how those relationships shape individuals’ viewpoints, as well as change the collective attitudes and behaviors of a population over time.
Studies such as those confirm a troubling spread of extreme polarization across the United States.
Two Arizona State University researchers and one of their colleagues have recently examined the roots of the trend toward accelerating social divisiveness through their computational lens.
Stephanie Forrest, a professor of computer science in the Ira A. Fulton School of Engineering at ASU and director of the Biodesign Center for Biocomputing, Security and Society, along with Joshua Daymude, a computer scientist and postdoctoral researcher at the center, and Robert Axelrod, a professor of political science at the University of Michigan, used agent-based modeling to connect individual behaviors to a population’s polarization. The goal was to gain insight about how polarization might be prevented.
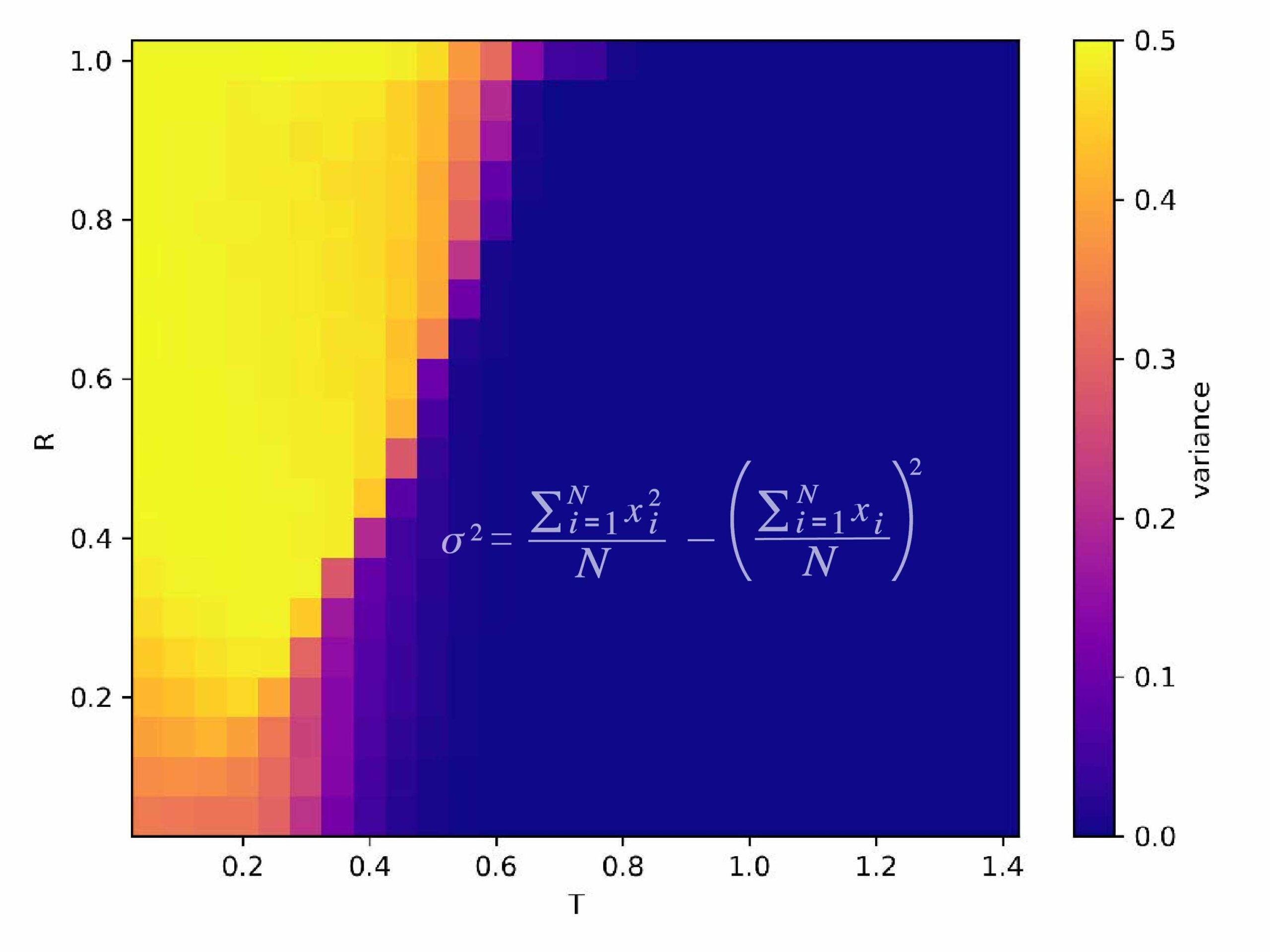
The yellow-to-blue phase diagram from the research paper “Preventing Extreme Polarization of Political Attitudes” by researchers Robert Axelrod, Stephanie Forrest and Joshua Daymude visualizes what their computational model predicts about political polarization as they increase “responsiveness” (moving up in the diagram) and “tolerance” (moving right in the figure). Yellow means that parameter value combination resulted in extreme polarization, while dark blue is the opposite (complete consensus). The intermediate colors are somewhere in between. The figure shows that the predictions of the model transition abruptly from extreme polarization to complete consensus as tolerance is increased.
The research team’s findings are reported in the paper “Preventing Extreme Polarization of Political Attitudes,” published in the Proceedings of the National Academy of Sciences.
In the paper, the authors articulate the threat of the societal fracturing that motivated their study: “Democracies require compromise. But compromise becomes almost impossible when voters are divided into diametrically opposed camps. The danger is that intolerance will grow, democratic norms will be undermined, and winners will be reluctant to let the losers ever regain power.”
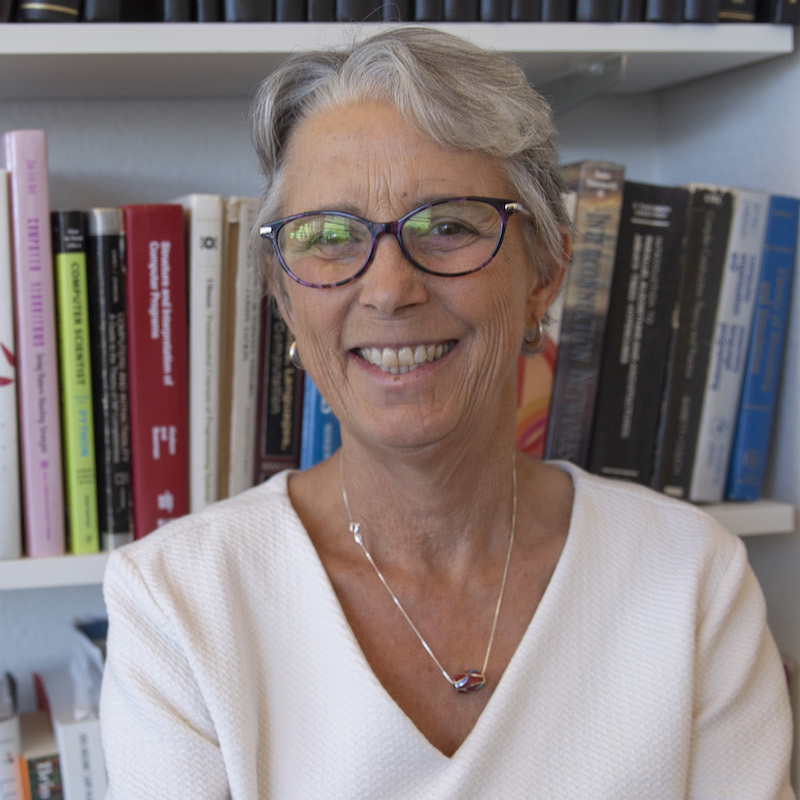
Professor Stephanie Forrest’s pursuits as a computer scientist include cybersecurity, software engineering, evolutionary computation and complex adaptive systems. Her work also focuses on the biology of computation and computation in biology, including biological modeling of immunological processes and evolutionary diseases. Photographer: Erika Gronek/ASU
Forrest, who teaches in the School of Computing and Augmented Intelligence, one of the seven Fulton Schools, has an external professor position with the Santa Fe Institute, which is a world leader in complexity science, focusing on problems and systems that are multidimensional, unpredictable and often rapidly changing, such as the polarization problem.
Forrest, Daymude and Axelrod sought to make their model as simple as possible so they could connect polarization’s causes and effects. Their Attraction-Repulsion Model has two simple rules: (1) people usually interact with others who are similar, and (2) interactions between similar people bring them closer together while interactions between different people push them further apart.
To adapt their computational approach to the sociological nature of the polarization problem, Forrest and Daymude drew on their experience in modeling how individual microscale actions within biological and technological systems produce various macroscale phenomena.
“We tried to make the simplest model possible that captures what we thought were realistic assumptions,” Daymude says. “It enables us to ask questions like what happens over time when people are more or less tolerant of others’ ideological positions, or more or less likely to be exposed to differing viewpoints.”
What they discovered contrasts sharply with the notion that opposites attract.
The researchers’ model confirms that a high level of intolerance between divergent groups is a key component of intensifying extremism and ideological polarization. They were surprised, however, to find that not all extremism made polarization worse.
“I think the number one surprise was finding that having a few rigid extremists in your midst can actually help establish and sustain a moderate majority,” Forrest says.
“Having a group that we can look at and say, ‘Oh, we don’t want to be like them, they are way out there on the edge politically’ can reinforce a centrist position among moderates,” Forrest says. “But in the absence of those extremists, those moderates may drift toward an extreme.”
For historical perspective, the researchers point to the period of civil unrest in the U.S. during the mid to late 1960s — during the Vietnam War era — when political dissent and societal schism led to strongly divided factions unwilling to find middle ground.
In that environment, much like today, groups on different sides of the issues siloed themselves from each other, creating “dangerous feedback loops” among adherents to specific ideologies, which fostered narrow and distorted views of others “that became more extreme overtime,” Forrest says.
But merely breaking groups out of their heavily fortified ideological shells isn’t always the solution.
“Our results suggest that simply exposing people to those with opposing views won’t make either side moderate their positions in the absence of other influences,” Forrest says.
Their findings suggest that when individuals are stubbornly intolerant, bringing people with divergent opinions together may not help them to bridge the proverbial divide — instead, it may make animosities more severe.
“While it at first may appear contrary to practical experience, our model suggests that strictly limiting exposure to dissimilar views could be an effective mechanism for avoiding rapid polarization,” Daymude says.
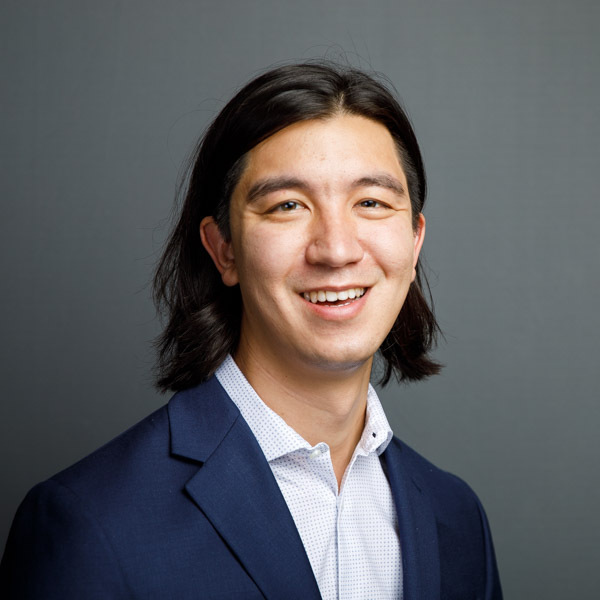
Joshua Daymude earned a doctoral degree in computer science from the Ira A. Fulton Schools of Engineering earlier this year. His research focuses on the algorithmic foundations of emergent collective behavior, using the theory of distributed computing and randomized algorithms to characterize how local interactions induce large-scale phenomena. Photographer: Andy DeLisle/ASU
The researchers point to efforts in the 1970s to accelerate desegregation in schools with busing as one historical example of how increased exposure can backfire. In places like Boston, busing exacerbated existing hostilities instead of fostering tolerance.
Other inferences drawn from the researchers’ computational model suggest interventions that might defuse levels of divisiveness.
When individuals are incentivized to adopt moderate positions — for example, by their economic self-interest or the news media they follow — polarization can be drastically reduced or avoided altogether.
“Even a very small amount of shared self-interest can dramatically reduce polarization,” Forrest says. “This is perhaps the most promising result of the computational modeling because it suggests a direction for policy intervention by which this polarizing dynamic could be moderated.”
Forrest says the project’s results don’t provide enough solid conclusions “to go to the policymakers and say ‘This is what you should do.’ But they do point to strategies that are more or less likely to succeed.”
The researchers still think that their results can be useful in formulating policies and mapping steps to effectively resolving differences to a meaningful degree.
“We want our findings to shed light on actionable steps we can take both at the level of policy and as individuals to understand and mitigate polarization before it compromises democracy,” Daymude says.
The idea for project that led to Forrest, Daymude and Axelrod’s paper evolved from discussions between ASU and Princeton University faculty members as part of an ASU Dialogues in Complexity Workshop led by Simon Levin and supported by ASU’s Office of the President. Levin is a professor in ecology and evolutionary biology and director of the Center for BioComplexity at Princeton University. The research paper is part of a PNAS special issue that arose from that project.
Support for the research came from the National Science Foundation, the Army Research Office, the U.S. Defense Advanced Research Project Agency and the Air Force Research Office.
Some content in this article was provided by William Ferguson, a writer for the Santa Fe Institute