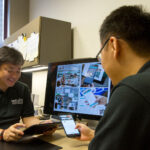
Human brain inspires self-learning microchip
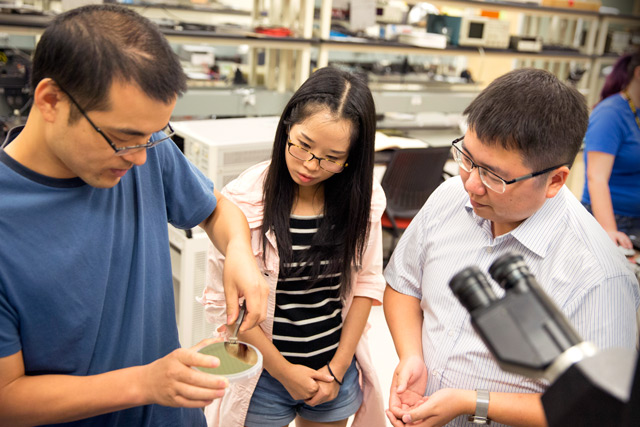
As part of his National Science Foundation CAREER award, Shimeng Yu (right) is providing interdisciplinary educational opportunities in neuro-inspired computing research for students in his lab. Pictured Rui Liu (center), an electrical engineering student, and Ligang Gao (left), an assistant research scientist. Photographer: Pete Zrioka/ASU
Neuroscience, microelectronics and computing — seemingly varied disciplines — have found a common intersection in the form of neuro-inspired computing.
This evolving field in computer engineering aims to emulate the human brain’s abilities for perception, action and cognition in our computer systems.
Neural systems provided inspiration for some of the earliest computing systems, but as the technology evolved it began to follow a different approach.
Assistant Professor Shimeng Yu is convinced that a radical shift back toward neuro-inspired architecture is needed in computer engineering.
This shift would break path with conventional methods — namely the von-Neumann architecture that has been used for the last 70 years — in order to offer faster processing and increased battery life.
Specifically, Yu aims to advance neuro-inspired computing by utilizing emerging nano-device technologies to create a self-learning microchip.
His research efforts are supported in part by a prestigious National Science Foundation CAREER award, which recognizes emerging education and research leaders in engineering and science.
A self-learning microchip
Yu’s microchip (a packaged unit of computer circuitry) boasts the capacity to learn things in near real time, adapt to its environment and make its own informed decisions, all while consuming much less power.
It can be embedded into mobile phones and sensor devices to perform certain intelligent tasks, making it useful in “a wide range of applications with profound consequences to our society,” says Yu.
The intelligent information processing paired with power-efficient mobile platforms could boost new markets in facial recognition software and self-driving cars.
It could also be used for security and defense applications, such as surveillance and identification of people and objects in real time.
If attached to frontend sensors, it could have “a groundbreaking impact on the internet of things from chemical and gas sensors to smart wearable systems used in social networks to personalized healthcare applications,” says Yu, adding that the battery lifetime of these devices can also be greatly extended.
Yu’s unique technical contribution involves pairing a crossbar array with resistive synaptic devices. In this system, orthogonal metal wires crisscross to form a crossbar array, and at each cross-point there is a resistive synaptic device, which is a resistor whose conductance (the degree to which it conducts electricity) changes by programming voltage pulses.
The change in conductance is what spurs learning in the chip, similar to how the human brain acquires and processes new neural information.
The resistive synaptic device emulates the properties of the synapses in a human’s brain. Synapses are small gaps at the end of neurons (nerve cells) that allow the neuron to pass an electrical or chemical signal to another neuron. When two connected neurons fire (become active), the conductance of their synapses changes, which causes new information to be learned.
Contributing to a radical shift
Yu’s discoveries are representative of a shift in the computing paradigm from von-Neumann computer architecture to neuro-inspired architecture. In today’s von-Neumann computer architecture, the processor and the memory is separate, and the processor fetches data from the memory through the data bus for processing.
However, in this method there is a memory wall problem for data-intensive applications due to limited bandwidth.
“The back and forth data transfer causes a bottleneck in performance and consumes the most energy in the system,” says Yu.
On the other hand, neuro-inspired architecture emulates the human brain by better localizing the process.
The human brain is its own distributed computing system with billions of neurons and synapses.
“Neurons are simple computing units and synapses are local memories, and they are massively connected in a neural network through communication channels,” explains Yu.
The neural data is stored in the synapses, which are close to the neuron units, making it easy for data to be locally fetched and processed by the neurons.
Simulating this neural process in computer architecture can eliminate the memory wall problem, or the data traffic jam, faced in the von-Neumann computer architecture.
As part of his CAREER award Yu is also integrating educational opportunities for students in his research efforts. He sees it as a perfect opportunity to train undergraduate and graduate students in interdisciplinary skills.
“The cross-layer nature of this research, ranging from semiconductor device and circuit design to electronic design automation and neuro-inspired machine learning, provides an ideal platform for preparing interdisciplinary students,” says Yu.
“The beautiful analogy between artificially fabricated synaptic devices and biological synapses in our neural systems has always fascinated me,” he says. This parallel will continue to drive Yu’s research and teaching efforts as he ushers in the next phase of computing architecture.
Media Contact
Rose Serago, [email protected]
Ira A. Fulton Schools of Engineering