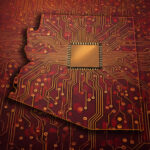
Machine learning makes wireless communication more efficient
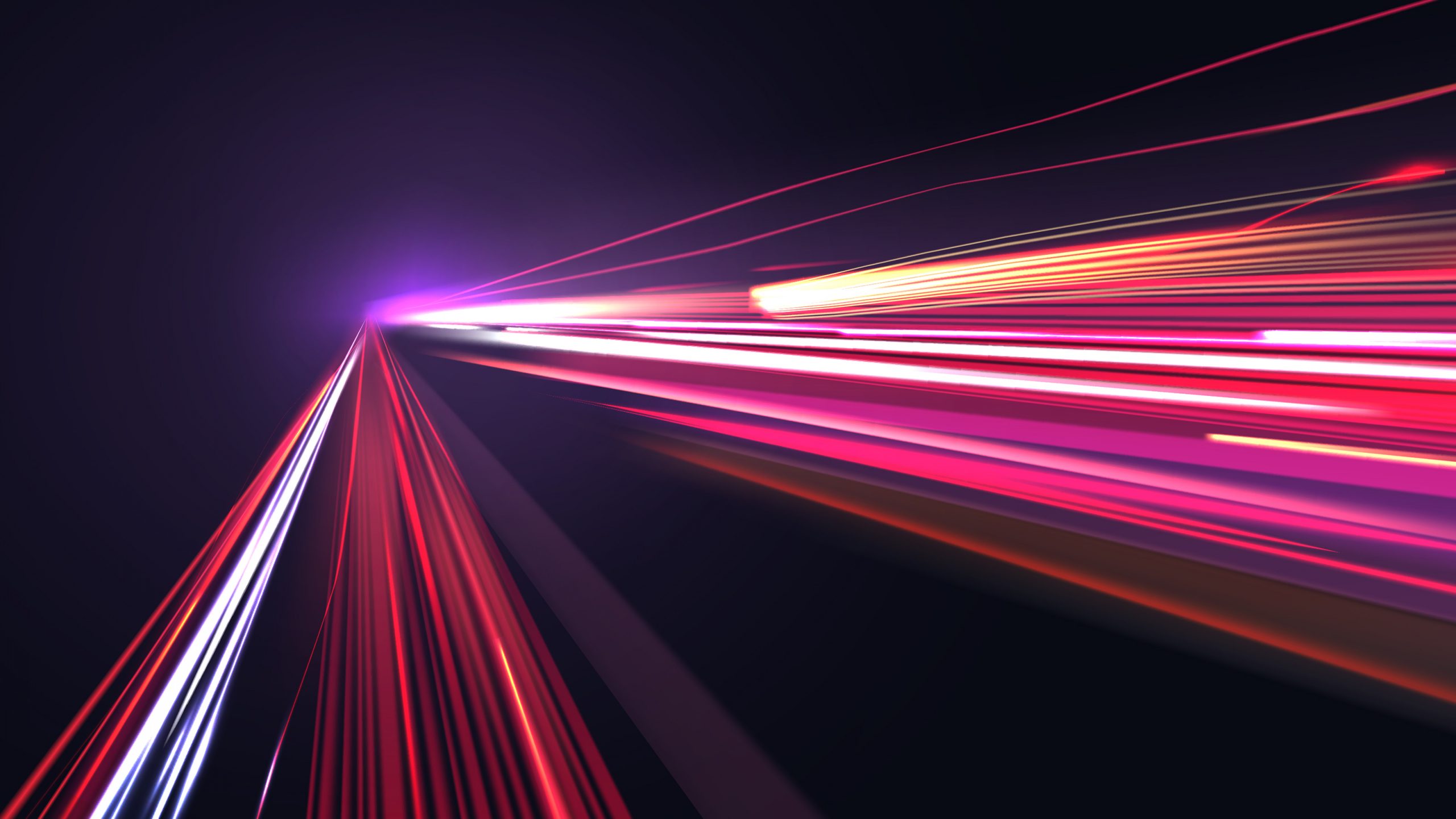
Above: Arizona State University Assistant Professor Ahmed Alkhateeb is implementing new machine learning approaches to help complex, large-scale wireless communication antenna systems more quickly and reliably predict channels and communication beams. This work, supported by a $500,000, five-year National Science Foundation Faculty Early Career Development Program (CAREER) Award, will help 5G and next-generation communication systems deliver more data to more devices, even when they’re on the move. Photo courtesy of Shutterstock
Nine faculty members in the Ira A. Fulton Schools of Engineering at Arizona State University have received NSF CAREER Awards in 2021.
While antennas may seem old-fashioned — think rabbit ears on television sets, appendages on mobile phones and aerials extended from cars — the antennas of today are less conspicuous but still very important for feeding all the data we crave to our wireless communication devices.
One of the best ways to keep up with the increasing demand for data is to add more antennas to communication systems, like emerging 5G wireless networks. Additional antennas can serve up more data at a faster rate, and support more devices in the same area.
“The problem, however, is that as we add more antennas to achieve these gains, we get a lot of challenges,” says Ahmed Alkhateeb, an assistant professor of electrical engineering in the Ira A. Fulton Schools of Engineering at Arizona State University. “The added complexity makes it very hard to support highly mobile communications systems like autonomous vehicles and wireless virtual or augmented reality with reliability and low latency (delay).”
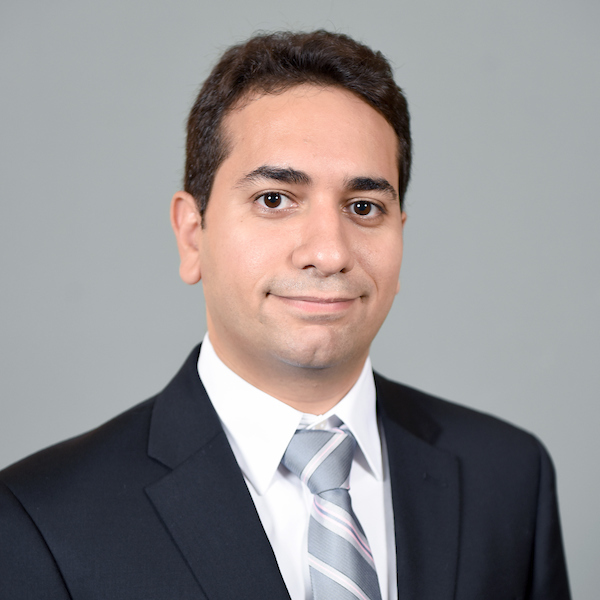
Ahmed Alkhateeb
To address this problem, Alkhateeb is working on ways to implement new machine learning approaches to cut through the complexity. The task is part of a research project supported by a five-year, $500,000 National Science Foundation Faculty Early Career Development Program (CAREER) Award.
Alkhateeb conducts research in the domain of multiple-input, multiple-output, or MIMO, communications systems, particularly large-scale MIMO systems that use large a number of antenna elements to transmit and receive signals with high data rates. Large-scale MIMO systems are already being adopted as key components of 5G communication infrastructure.
This type of communication requires what is known as beamforming — which is when a base station like a cellular tower and a device like an iPhone both focus a tight, directional, high-frequency signal beam to each other to make a data connection, like a handshake.
To make this connection, the large-scale MIMO system must estimate a channel, or directional angle, through which the beams can connect. This requires the transmitter to know some information about the signal propagation over the air to the receiver. This propagation implicitly relies on the location of the receiving antenna and details of the environment, such as buildings, that surround the transmitter and receiver.
Channel estimation for massive numbers of antennas is complex and time-consuming, but it is especially critical for fast-moving targets, such as communications in autonomous vehicles.
“If we estimate the channel, but the target moves, we need to estimate the channel again and we may not have enough time to actually transmit data,” Alkhateeb says.
That’s where the addition of machine learning techniques can help.
“We are relying on machine learning to predict statistical information about the channel that will be used later on by the classical [signal processing] system to estimate the channel in a robust and efficient way,” he says. “We can leverage prior observations and side information such as positions and camera images to support more mobility, reliability and lower latency.”
For instance, 5G systems use both low-frequency and high-frequency bands of the electromagnetic spectrum for communication. When a lot of data needs to be sent with little delay, high-frequency bands are more desirable but adjusting beamforming at these bands is difficult to perform quickly.
“We can estimate the channel at low frequency within a very short time and use it to predict a channel at high frequency that typically requires long training overhead,” Alkhateeb says. “So, we map the channel from one band to another and by doing that we can save a lot of time.”
He is also using additional information, such as the position of base stations or mobile devices and visual data from cameras, to enable awareness of the user’s location and the geometry of the environment. This awareness can more quickly and easily guide channel estimation.
Adding cameras to base stations and using existing cameras, for example in autonomous vehicles, can provide more awareness that can speed up channel estimation.
“The base station will visually see where the vehicle is, and the vehicle can characterize where the base station is, and that can at least reduce the search space,” Alkhateeb says. “Instead of searching everywhere, it at least knows to search in particular directions.”
In addition to developing algorithms for machine learning to help with the channel estimation process, Alkhateeb is working with undergraduate electrical engineering students to build hardware proof-of-concept prototypes to demonstrate the technology in practice.
“This research can show that by using machine learning, we can significantly reduce the time and complexity overhead of the channel estimation and beamforming design,” he says. “This will, hopefully, enable large-scale MIMO in future wireless communications systems and will allow these systems to support applications with mobility, strict reliability, low latency and high data rate requirements.”
His team will make data sets that can help advance machine learning research in the wireless communications space, which is a relatively new area of study.
And because Alkhateeb is developing solutions that take into consideration the practical limitations of actual wireless communication hardware and systems, he is hoping the solutions he and his research team develop will be feasible to put into practice in the near future.