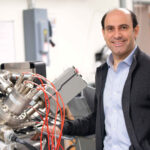
Machine learning gets interactive for better data-driven impact
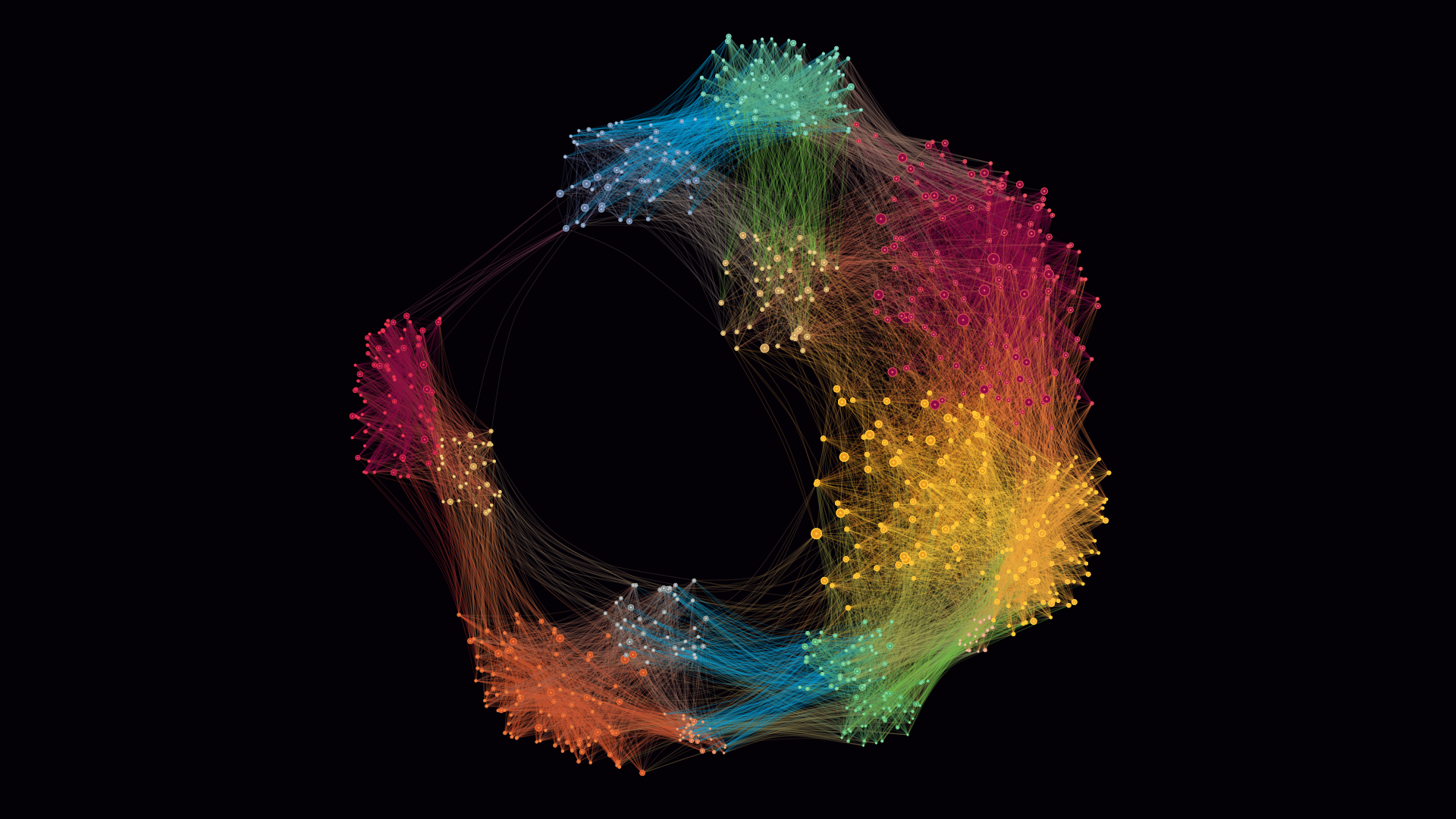
Above: Machine learning and data science capabilities for analyzing large, complex networks don't currently meet expectations for accessibility and efficiency. Arizona State University Assistant Professor Gautam Dasarathy is developing interactive machine learning algorithms in a new NSF CAREER Award project to increase the effectiveness of data analysis for many real-world applications. Graphic by Rhonda Hitchcock-Mast/ASU and Shutterstock
Nine faculty members in the Ira A. Fulton Schools of Engineering at Arizona State University have received NSF CAREER Awards in 2021.
Large, complex, interacting systems are everywhere around us — from the synapses in our brains to the internet. These systems are often modeled as graphs or networks, which are essentially a collection of objects and the connections among those objects.
“Graph-based algorithms are crucial for a wide array of modern technological and scientific achievements such as the reliable operation of the power grid and the internet, or the understanding of the human brain,” says Gautam Dasarathy, an assistant professor of electrical engineering in the Ira A. Fulton Schools of Engineering at Arizona State University.
“Given our rapidly expanding ability to collect and crunch data,” he says, “the moment seems ripe to take on several important problems in science, engineering and society using this powerful methodology.”
But there’s a catch. Dasarathy says that there is a “stark disconnect between the promised capabilities of machine learning and data science on graphs and their real-world applicability.”
Meaningful use of graphs often requires expensive testing and measuring, vast amounts of time, or a high level of expertise in acquiring curated data unavailable to most. These problems get worse as the graphs get larger and more complex.
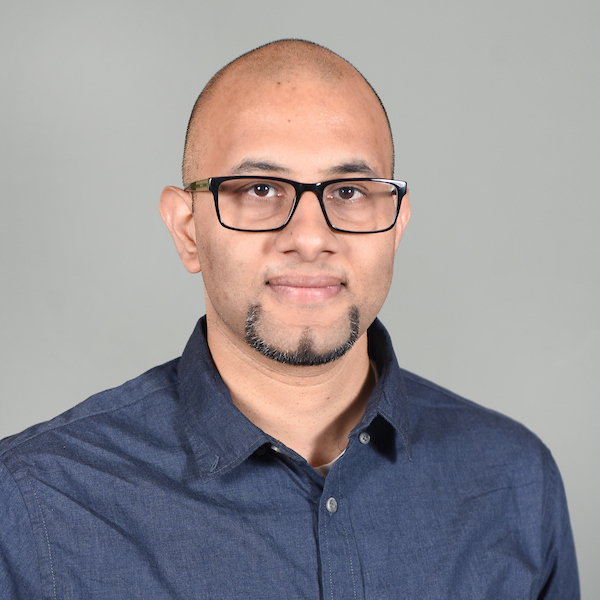
Gautam Dasarathy
Dasarathy is rethinking traditional machine learning and information processing by developing interactive machine learning algorithms in a new project, “Learning and Leveraging the Structure of Large Graphs: Novel Theory and Algorithms,” funded by a 2021 National Science Foundation Faculty Early Career Development Program (CAREER) Award.
CAREER awards are granted to early career researchers who are potential leaders in their field. This award provides Dasarathy $600,000 over five years to support his work.
“I feel fortunate that my proposal found a [review] panel that saw some promise in my research vision,” Dasarathy says.
An important feature of Dasarathy’s new machine learning algorithms for graph structures is that they will sequentially and adaptively decide what data they need to learn and perform their assigned tasks.
“Instead of passively crunching pre-collected data,” Dasarathy says, “these new algorithms will actively participate in the data acquisition process, thereby enabling significantly greater efficiency and effectiveness.”
He will also explore how the algorithms can be used for generating efficient inferences and decision making, as well as study the tradeoffs between data acquisition costs and statistical accuracy.
This advancement in machine learning for graphs is needed for many real-world applications: prediction and preemptive intervention during pandemics, management and utilization of resilient cyber-infrastructure, and state-of-the-art neuroimaging and wireless communication.
“I am particularly excited because the theoretical and methodological advances I will develop as part of this project will complement and directly contribute to a number of collaborations I have developed with researchers at ASU and beyond,” Dasarathy says.
He is currently working on several projects funded by the NSF and National Institutes of Health that would benefit from better data-driven approaches. These collaborations include multidisciplinary research teams from the School of Electrical, Computer and Energy Engineering and the School of Computing, Informatics, and Decision Systems Engineering — two of the six Fulton Schools — as well as the ASU School of Arts, Media and Engineering and School of Geographical Sciences and Urban Planning.
“This ability to widely collaborate with domain experts from various disciplines is frankly the one thing that gets me up and going every morning,” Dasarathy says, adding a sentiment inspired by statistician John Tukey: “I am thrilled that I get to play in everyone’s backyard.”
The “culture of open collaboration and generous mentorship” was a major factor in Dasarathy’s decision to come to ASU in the fall of 2018. The working environment and the strong research support the Fulton Schools has provided is enabling Dasarathy to build a research program around questions that inspire him and to pursue use-inspired fundamental research.
“We are at a juncture where the scientific and engineering communities are in desperate need of novel ideas to truly harness the data revolution,” says Dasarathy, referring to one of the NSF’s 10 big ideas. With this project, he hopes to make meaningful and long-term impact toward satisfying this need.
As part of the NSF CAREER award project, Dasarathy will also integrate education, mentoring and outreach related to his research to increase student awareness, access and inclusion in developing data-driven methods needed to solve big societal challenges.