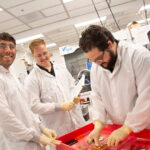
Li receives CAREER Award for transfer learning research
Jing Li, assistant professor in industrial engineering in the School of Computing, Informatics, and Decision Systems Engineering, has received a CAREER Award for her work developing transfer learning methodologies to help improve manufacturing systems and healthcare.
The notion of transfer learning was originally introduced in psychology and education. It refers to the study of how humans apply prior experience to learn new tasks.
Li is the first to introduce transfer learning into the area of quality control and process improvement. In this new context, transfer learning refers to gaining knowledge from one quality control process then applying that knowledge to a different but related problem.
In statistical quality control, statistical models are built from production data to characterize the relationships between many process and product quality variables for process monitoring, fault root cause identification and quality control decision makings. Finding ways to build these models is more complex today, with many industries—in particular semiconductor and renewable energy—facing challenges of high product variety and short life cycles. A development path that used to be several years is now just several months.
“In this scenario, the traditional quality control paradigm falls short,” Li says.
“Now we need to use a very limited amount of data and knowledge, and a short time period of learning. Because
the data is so limited, we cannot get a reliable statistical model that can be used for the new generation of products.”
Li’s approach is to leverage information that is embedded in old processes—for example, processes that share the same production environment, same machines or same product family. The objective is to use prior information as a baseline, then focus on quickly learning the new product.
One of the challenges is that existing data cannot be used directly—simply pooling the old data with the new data will not work as the production processes are not identical. Her work will develop novel transfer-learning-based methods with statistical rigor and computational efficiency, to address various quality control objectives including process monitoring and anomaly detection, fault root-cause analysis and quality control with sensor data uncertainty.
By enabling real-time, robust quality control decision-making across a large variety of products and along progressing product generations, the results of this research could significantly improve the quality, productivity and cost-effectiveness of manufacturing systems. The potential for Li’s research is not limited to industrial processes. She is also exploring applications in healthcare. Using neuroimaging data for patients with similar neurological disorders, transfer learning can help identify differences in advanced phases of diseases such as Alzheimer’s disease, and provide better diagnosis and treatment options throughout the progression of the disease.