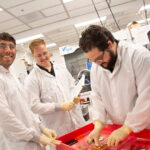
Artificial intelligence applications for a more resilient power grid
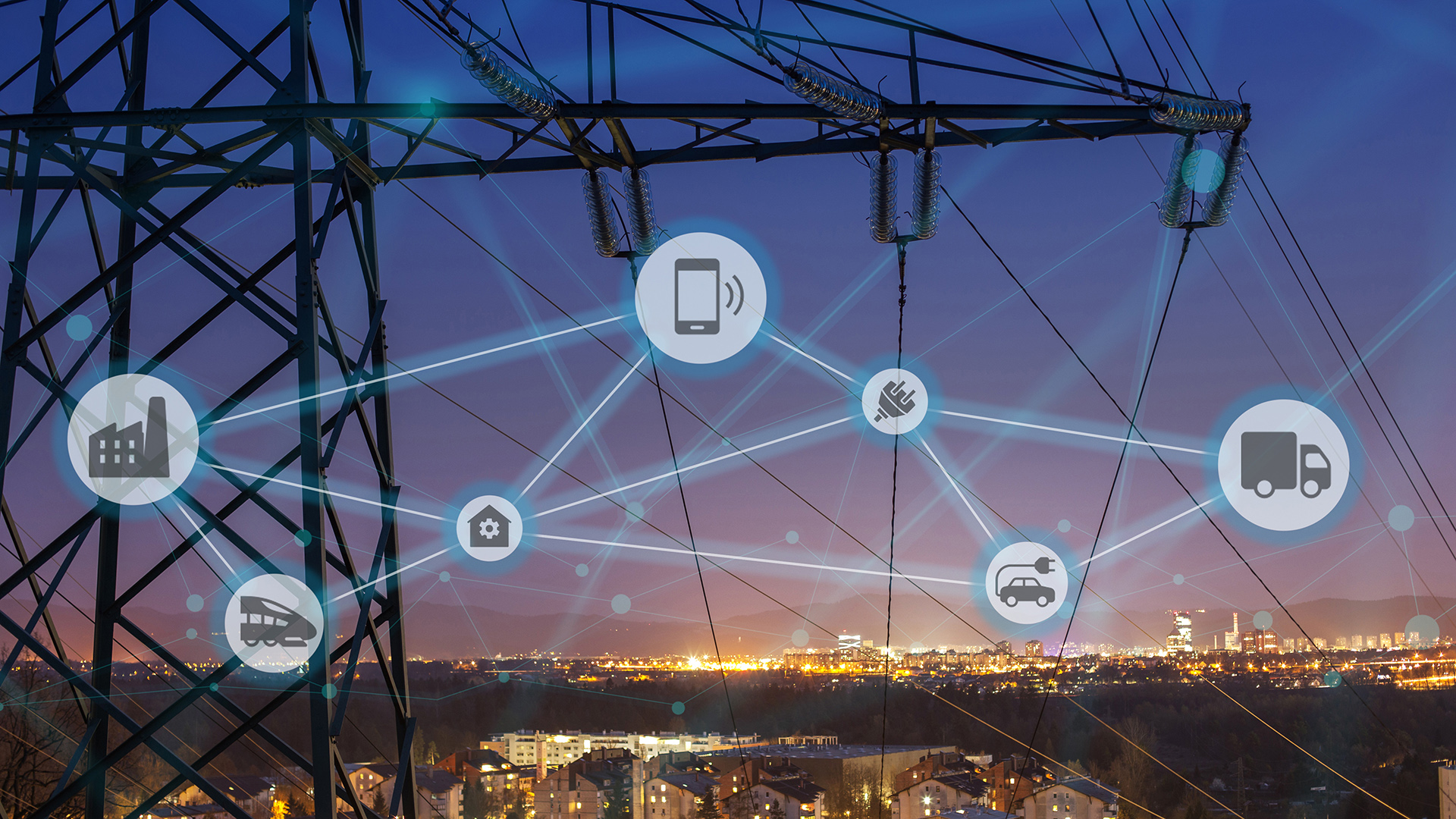
Above: Yang Weng, an assistant professor of electrical engineering, will be using machine learning and artificial intelligence to create more robust mathematical models to assess power flow in the electrical grid in areas without sensors collecting real data. Photo courtesy of Shutterstock.
Nine faculty members in the Ira A. Fulton Schools of Engineering at Arizona State University have received NSF CAREER Awards in 2021.
Yang Weng, an assistant professor of electrical engineering in the Ira A. Fulton Schools of Engineering at Arizona State University, received a 2021 National Science Foundation Faculty Early Career Development Program (CAREER) Award to develop a theoretical foundation for a distribution grid for unobservable systems that will be more reliable under increased power flow.
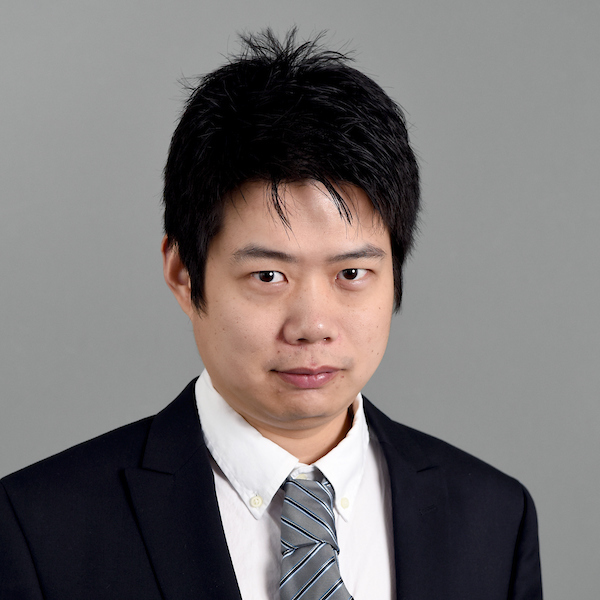
Yang Weng
“If a system is observable, there are working sensors in the area so that we can infer the physical laws behind it,” says Weng, who teaches in the School of Electrical, Computer and Energy Engineering, one of the six Fulton Schools. “When there is no sensor in one area, we call this area unobservable. My project will tackle the energy flow in the unobservable area with an artificial neural network for optimal approximation in this area.”
Weng’s project advances electric distribution grid technology in three primary ways, all of which focus on unobservable areas of the electric distribution grid.
The first of these aims is to develop and deploy a deep neural network, or DNN, which uses machine learning and artificial intelligence to generate artificial data to create power flow equations that can represent the unobservable areas of the electric distribution grid.
In observable areas, sensors collect real-time data that can be used to generate equations to describe the power flow behavior. With the DNN generating artificial data in unobservable areas, theoretically new power flow equations can be generated to design machine learning algorithms.
That leaves a mathematical gap between how the system is modeled using real data instead of artificial data.
Weng plans to design the neural network so that it can also translate to areas of the electric grid that are observable using sensors and exhibits behaviors that can be described by physical laws.
“When one designs machine learning algorithms, one common trouble is that the learning result may not be optimal due to using artificial approximation,” Weng says. “Therefore, one natural question is if we can design the DNN for artificial approximation, but let machine learning reduce to physical laws. This is one of the biggest novelties in the project.”
The second aim of the project is to create a physical-generative adversarial network that can boost the robustness of modeling power flow with the limited data provided by unobservable areas.
A generative adversarial network is a structure composed of multiple deep neural networks that creates new artificial data. The generative adversarial network becomes a physical-generative adversarial network when it is used to generate artificial data that follows physical laws. This is a key component in bridging the mathematical gap between the unobservable areas and the observable areas.
The final aim of Weng’s project is to derive the solution for inverting the DNN-based power flow equation using the physical DNN for real-time power flow analysis to bridge the gap between the artificially generated power flow equations and the data-based power flow equations.
“This work opens the door for maximizing the profit derived from machine learning tools by mining the knowledge hidden behind everyday data and physical knowledge,” says Weng. “In the long term, it will create a new framework for accurate, fast and efficient algorithms for power system analysis with plug-and-play components and distinct behaviors.”